Remote identity verification is impossible without a proper image capture. But retaking the image to meet the requirements—set for your safety, by the way—is still very annoying. Fixing that problem is one of the major purposes of using neural networks in mobile apps.
Why you can’t just add neural networks to an app
In identity verification, neural networks allow you to assess (or even improve) the quality of images right on the spot instead of sending data back and forth from the device to the server and back again. In other words, they make the capture process really smart. Such functionality comes at a price though: neural networks add to the size of an app.
Say you’d like to implement facial recognition technology. A user opens the app, takes a selfie, and—voilà—gets approval to access a product or service. Behind the curtains, however, this task requires numerous neural networks. Speedy face detection, recognition, and face quality verification from the example above might need around 20 neural networks. The number can be even bigger, as the process requires checking and validating multiple parameters.
In the best-case scenario, each of these networks is about two megabytes. Simple multiplication shows that in this case, you’ll need about 40 megabytes of smartphone memory dedicated to storing the neural networks for a single app alone.
At the same time, there’s hardly a person who uses just one app on their smartphone. For example, an average user in the USA interacts with over 46 apps per month.
However, it’s not only the technical limitations of devices. Weighty apps—both mobile and web—tend to load longer, harming the user experience from the very start. Add to that an unstable internet connection, and you’ll witness a drastic fall in conversions and an increase in churn rate.
That’s why developers put a lot of effort into making neural networks (and apps in general) smaller yet effective.
Why neural networks are huge
For starters, how big is big? GPT-3, the already outdated version of a neural network model for creating all sorts of text, had over 175 billion machine learning parameters, requiring 800 gigabytes to store. While OpenAI, its developers, won’t say how much bigger the next-gen GPT-4 is, there are testimonies that the latest language model has 1 trillion parameters.
The example above is surely an extreme case. The networks that can come in handy for identity verification aren’t that huge. For example, CRAFT, which is an advanced text detection model, takes about 100MB of space. That’s still too much for an app, however.
So why are neural networks so large? The answer lies in the training process that enables them to fulfill their functions properly. Simply put, an operator feeds the network with large amounts of sample data.
Although there’s no fixed rule on the minimum training dataset size, the ten times rule is often used as a starting point. If, for example, an algorithm recognizes ID documents based on 1,000 parameters, you need at least 10,000 various document images to train the model. Face recognition may require even more samples: to prove someone’s identity via face matching, a neural network has to learn to differentiate millions of human faces.
The idea behind the process is simple: the higher the number of parameters in a neural network, the better its performance. Unfortunately, this is a double-edged sword, since more parameters at the same time slow down the inference—the delivery of output—and increase computational costs.
Just like with a human brain, a neural network encompasses both required and redundant neural connections after being trained. The latter either aren’t used at all, or duplicate active connections. However, the redundant connections don’t vanish: they remain deep inside the network, adding to its overall size.
Fitting all those neural network capacities into a limited smartphone storage space is quite a quest, but neural network compression techniques come to the rescue.
Neural network compression methods
Once a neural network is properly trained, the next stage is finding the right balance between efficiency and size. It’s a crucial task, as many systems where a neural network will be deployed have memory limits. The list includes not only mobile platforms, as web solutions are memory-sensitive too.
There are several methods of neural network compression that address two key parameters: time for network training and inference time. Below, we’ll dive into three of them that are used in Regula’s solutions for identity verification.
Weight pruning
Pruning is a neural network compression method used to cut off redundant neural networks’ parameters (weights) and eliminate all the excessive connections that the network stores inside. As biases are removed, the neural network’s size becomes smaller and starts to respond faster. As a result, you get an accurate neural network that takes up only as much device memory as it needs for correct functioning.
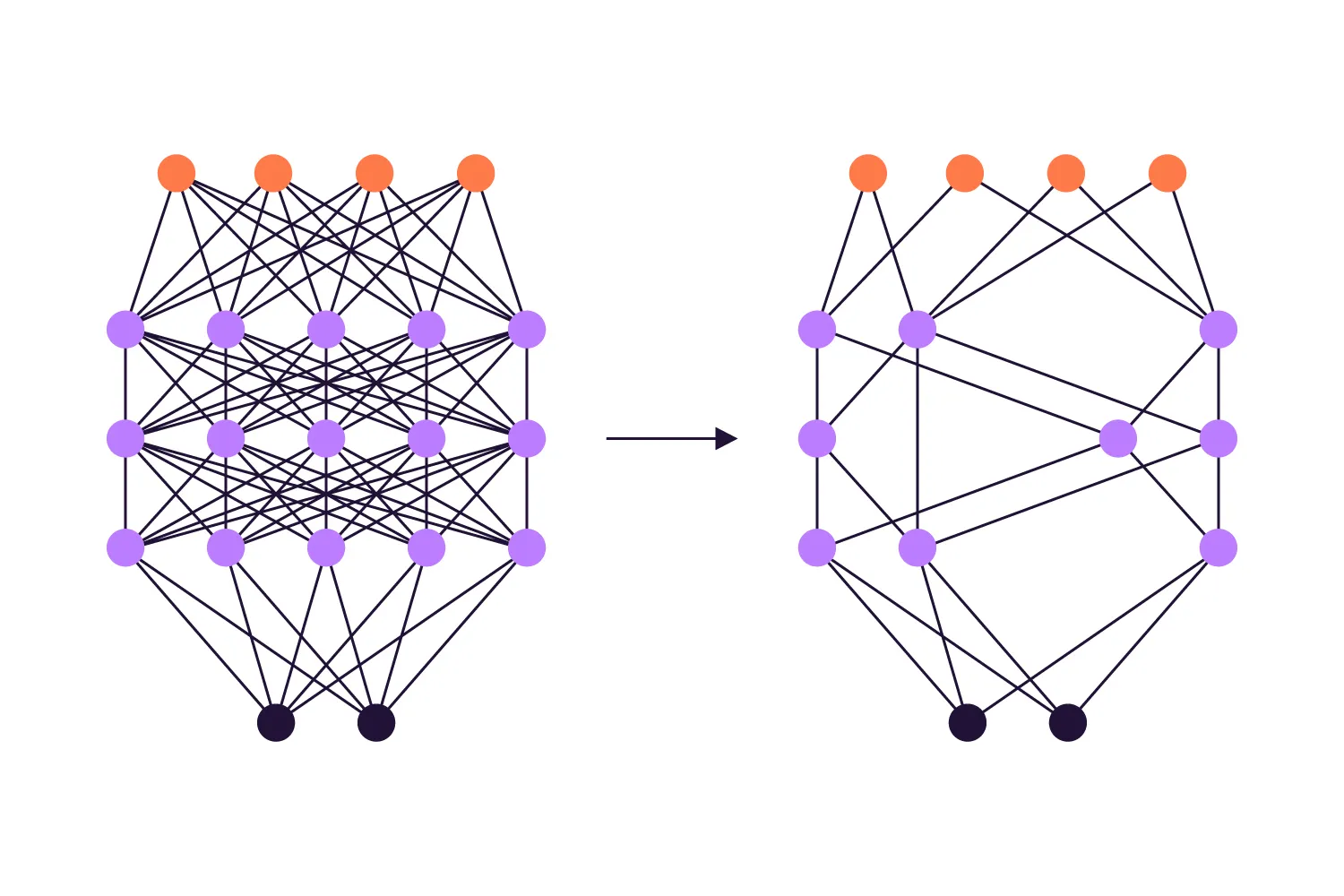
The weight pruning procedure seems to be quite the opposite of what happened at the training stage. Still, you don’t want the network to lose everything it learned. That’s why the “optimal brain damage” concept is applied. According to it, you need to remove as much as possible, but stop right before the performance starts to suffer.
The method involves numerous iterations:
Training the network
Estimating the importance of each weight
Removing the weight with low importance
Comparing the output to the initial via a feedback loop
Retraining the network
Going back to step 2
As good as it sounds, pruning alone is still not enough to make any neural network easily fit any platform, including mobile ones. That’s why we at Regula stop pruning our neural networks when their capacity and speed are the highest possible, regardless of the size they are at that moment, and go to the next stage.
Quantization
Neural network quantization is technically a process of reducing the precision of the network’s weights, for example, replacing floating-point numbers (like 1.3333…) with integers (133/100). The point is to decrease the variation of weights in the network, which is generally pretty high, and make them more similar to each other.
The logic of “making them more similar” derives from archiving algorithms: the lower the variation of data in a file, the larger the compression ratio. A text file containing only one repeating letter will be compressed with a higher ratio than a file containing various letters. The same principle applies to compressing neural networks, where more homogeneous weights allow for more efficient compression.
To make the quantization even more effective, we group similar weights into clusters. This clusterization leads to a significant entropy reduction. As a result, a neural network can be effectively compressed, even with an ordinary archiver.
However, with quantization, it’s relatively easy to lose the accuracy of a network. So in most cases, it’s a sort of trade-off between quality and size. But not for Regula.
The secret ingredient: Regula’s unique in-house methods of quantization
The AI industry knows that four- or five-fold compression—generally from 32 to 8 bits per weight—through quantization doesn’t significantly impact the accuracy and quality of neural networks. Yet, even a five-times compressed neural network won’t fit a mobile solution, at least for identity verification, since it requires tens of neural networks to operate.
In some cases, this would be the end of the story. Regula’s engineers, however, came up with unique in-house methods of quantization that make 10- and 14-fold compression possible without compromising quality. For example, the neural network that works as a face detector in Regula’s solutions can be compressed 10-12 times. The network for checking the holograms can become 12-14 times smaller in size.
And that’s not even the limit.
For some neural networks, the company’s experts have managed to attain a degree of compression from 23x to 32x. That means that every weight in a network—there are usually millions of weights in every single neural network Regula uses—can be reduced from 32 bits (the maximum) to 1 bit (the minimum).
Thanks to such breakthroughs, Regula’s SDKs for document and biometric verification can be integrated with a mobile and/or web app without bloating its size. Moreover, as new tasks arise, new networks can be added. That’s possible due to the ability to compress them many times without losing accuracy or speed, and without making a noticeable impact on the device or system memory.
To sum up
In the identity verification field, all major tasks, such as document or biometric recognition, verification against specific parameters, liveness checks, and more, are delivered by neural networks. Thanks to this, verification operations take seconds, are accurate, and allow you to cut operational costs.
Still, fitting neural networks into mobile or embedded solutions without sacrificing quality is always an engineering challenge. Fortunately, however, it’s one that the engineers at Regula managed to solve quite elegantly. If you are facing the task of deploying effective identity verification in your app, we’ll be more than happy to help you.